This course is organized in three main chunks. In the two weeks, we will discuss some core tensions that run throughout the course. In the next 5 weeks, we will learn about predictability–and its limits—in different substantive domains. Then, we will spend the remaining 5 weeks on themes that cut across different substantive domains.
Class slides are available to enrolled students via the Ed Resources page.
Monday, January 29: Introduction (Matt and Arvind)
No required reading before class.
Wednesday, January 31: Background (Arvind)
Monday, February 5: Why do we make predictions? (Matt)
Wednesday, February 7: Ethics (Arvind)
- Pre-read
- Johnson, R.A., & Zhang, S. (2022). “What is the Bureaucratic Counterfactual? Categorical versus Algorithmic Prioritization in US Social Policy,” Proceedings of the 2022 ACM Conference on Fairness, Accountability, and Transparency, 1671–1682.
- Wang, A., Kapoor, S., Barocas, S., & Narayanan, A. (2024). “Against predictive optimization: On the legitimacy of decision-making algorithms that optimize predictive accuracy,” Journal of Responsible Computing.
- Obermeyer et al. (2019). Dissecting racial bias in an algorithm used to manage the health of populations. Science.
- Benjamin. (2019). Assessing Risk, Automating Racism. Science.
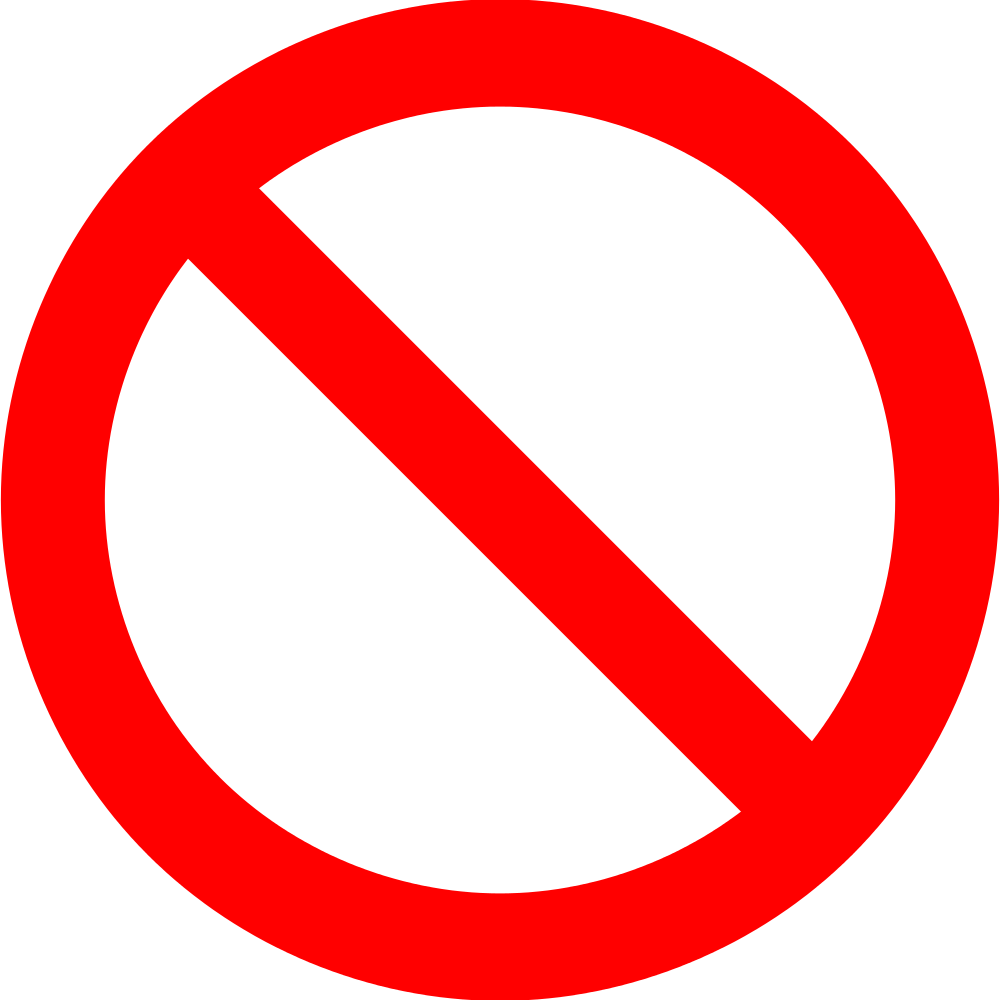
- Angwin, Larson, Mattu, Kirchner. (2016). Machine bias. ProPublica.
- Angwin & Larson. (2016). Bias in Criminal Risk Scores Is Mathematically Inevitable, Researchers Say. ProPublica.
Monday, February 12: Geopolitics (Matt)
- Pre-read
- Tetlock (2005) Expert Political Judgement: How Good Is It? How Can We Know?:
Wednesday, February 14: Geopolitics, part 2 (Matt)
Monday, February 17: Weather (Matt)
Wednesday, February 19: Weather, part 2 (Matt)
Monday, February 24: Cultural products and careers (Arvind)
- Pre-read: available to enrolled students on the Ed Resources page.
- Hedstrom (2006) “Experimental Macro Sociology: Predicting the Next Best Seller”, Science.
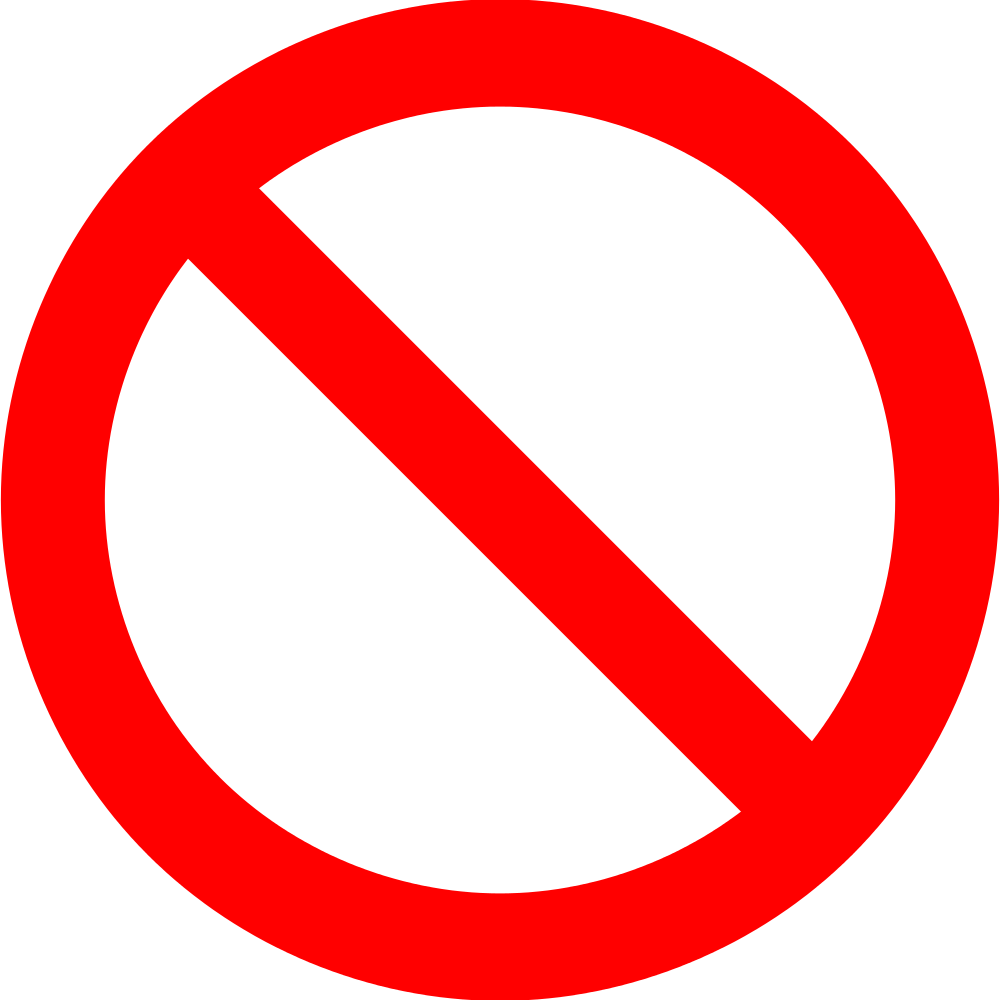
- Salganik, Dodds, Watts (2006) “Inequality and unpredictability in an artificial cultural market”, Science.
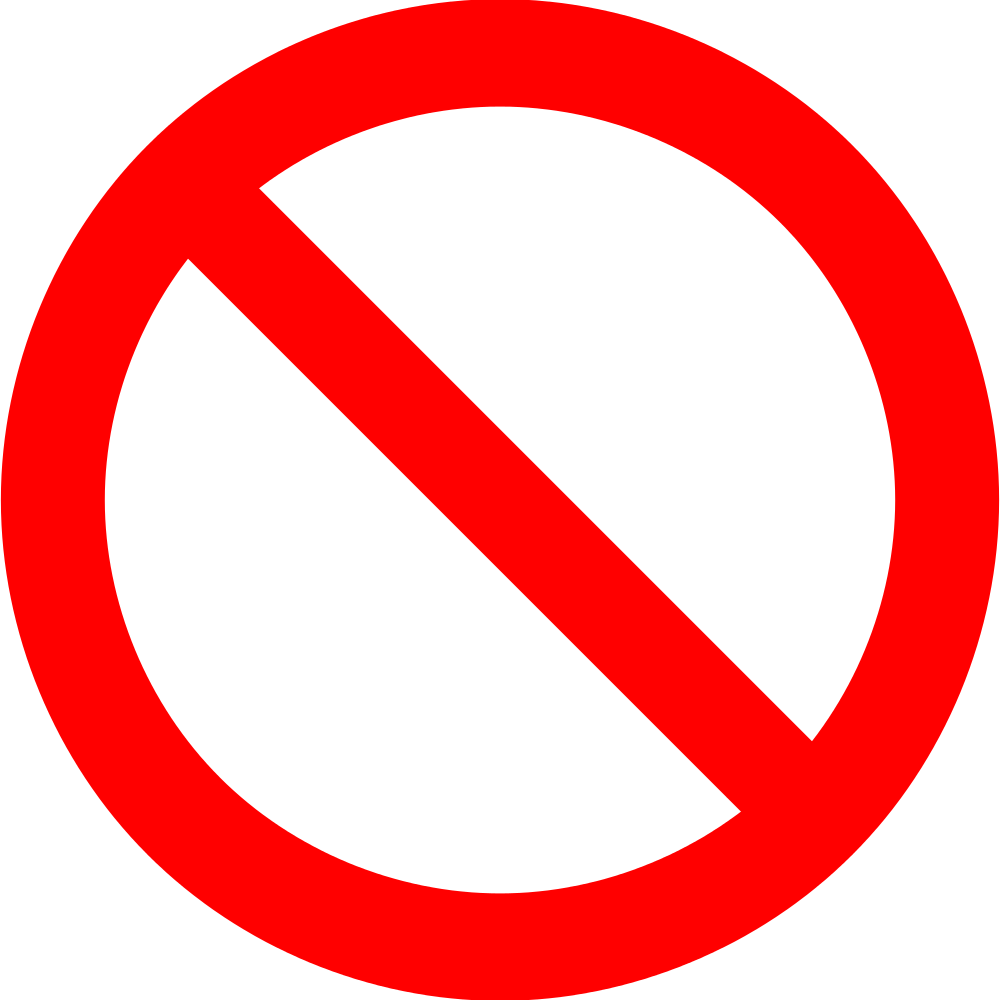
- Frank (2016) “Success and luck : good fortune and the myth of meritocracy”
Read chapter 2, chapter 3, and appendix 1.
- Goel, Hofman, Lahaie, Pennock, Watts (2010) “Predicting consumer behavior with Web search”, PNAS.
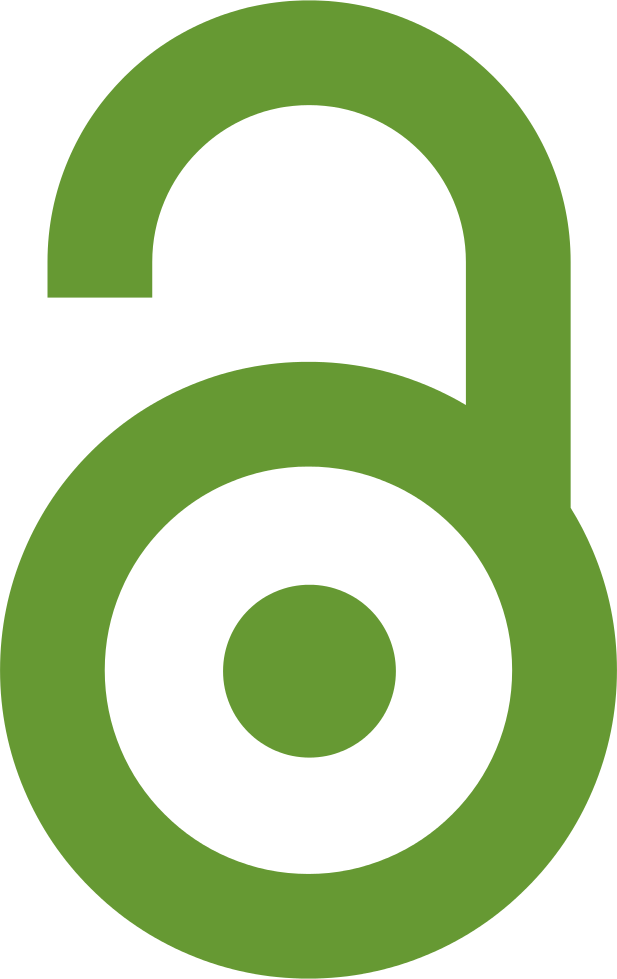
- Pre-read: available to enrolled students on the Ed Resources page.
- Narayanan (2023). “Understanding Social Media Recommendation Algorithms” Knight First Amendment Institute. Read only the first six sections, up until “Viral Content Dominates Our Attention”.
- Martin, Hofman, Sharma, Anderson, Watts (2016) “Exploring Limits to Prediction in Complex Social Systems”, WWW.
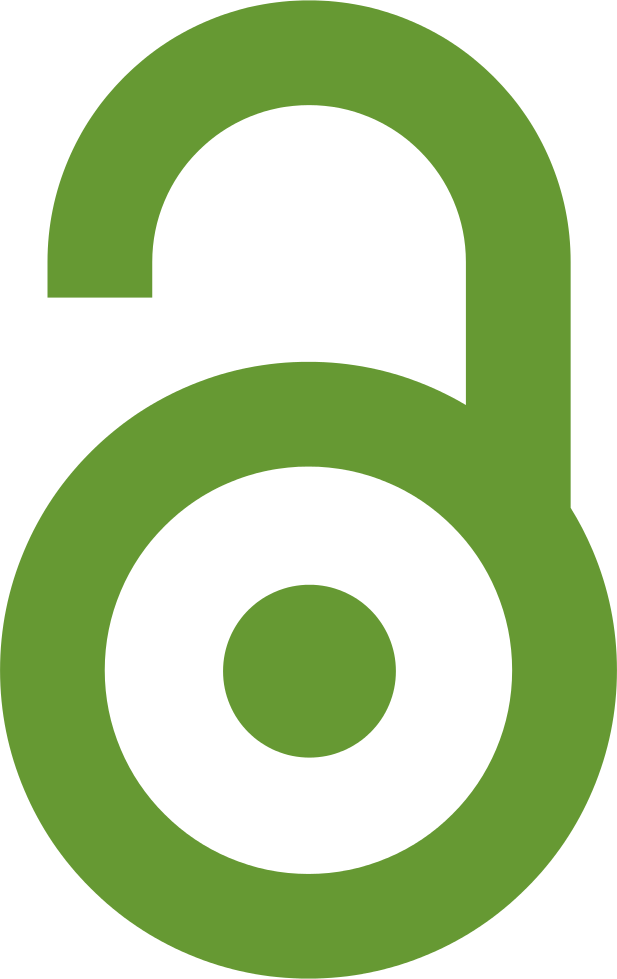
- Cheng, Adamic, Dow, Kleinberg, Leskovec (2014) “Can cascades be predicted?”, WWW.
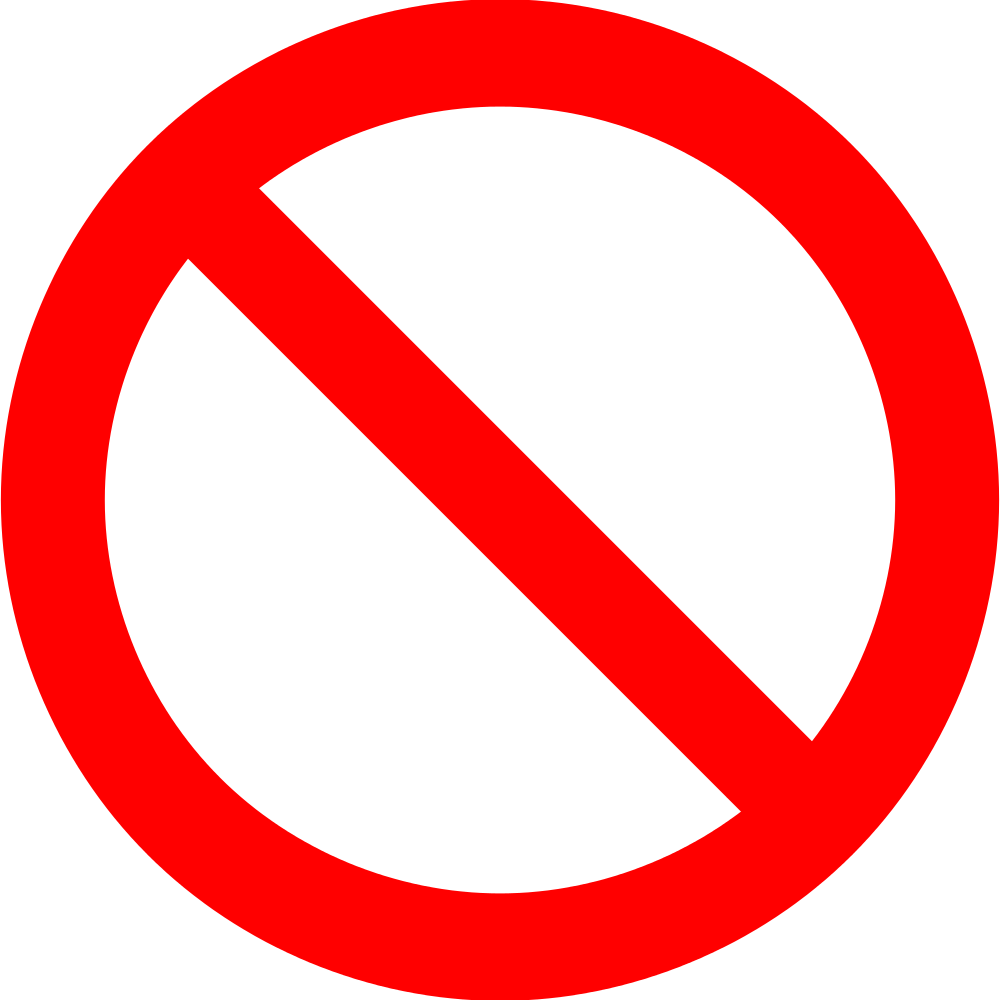
- Duffy, Pinch, Sannon, Sawey (2021) “The Nested Precarities of Creative Labor on Social Media” Social Media + Society.
Monday, March 4: Can advanced AI help predict the future? (Arvind)
- Pre-read
- Bommasani, R., Hudson, D. A., Adeli, E., Altman, R., Arora, S., von Arx, S., Bernstein, M. S., Bohg, J., Bosselut, A., Brunskill, E., et al. (2021). “On the opportunities and risks of foundation models.” arXiv preprint arXiv:2108.07258. Read only the Introduction.
- Lee, T. B., & Trott, S. (2023). “Large language models, explained with a minimum of math and jargon.” Understanding AI, 27, 2023.
- Savcisens, G., Eliassi-Rad, T., Hansen, L. K., Mortensen, L. H., Lilleholt, L., Rogers, A., Zettler, I., & Lehmann, S. (2023). “Using sequences of life-events to predict human lives.” Nature Computational Science, 1-14. Nature Publishing Group US, New York.
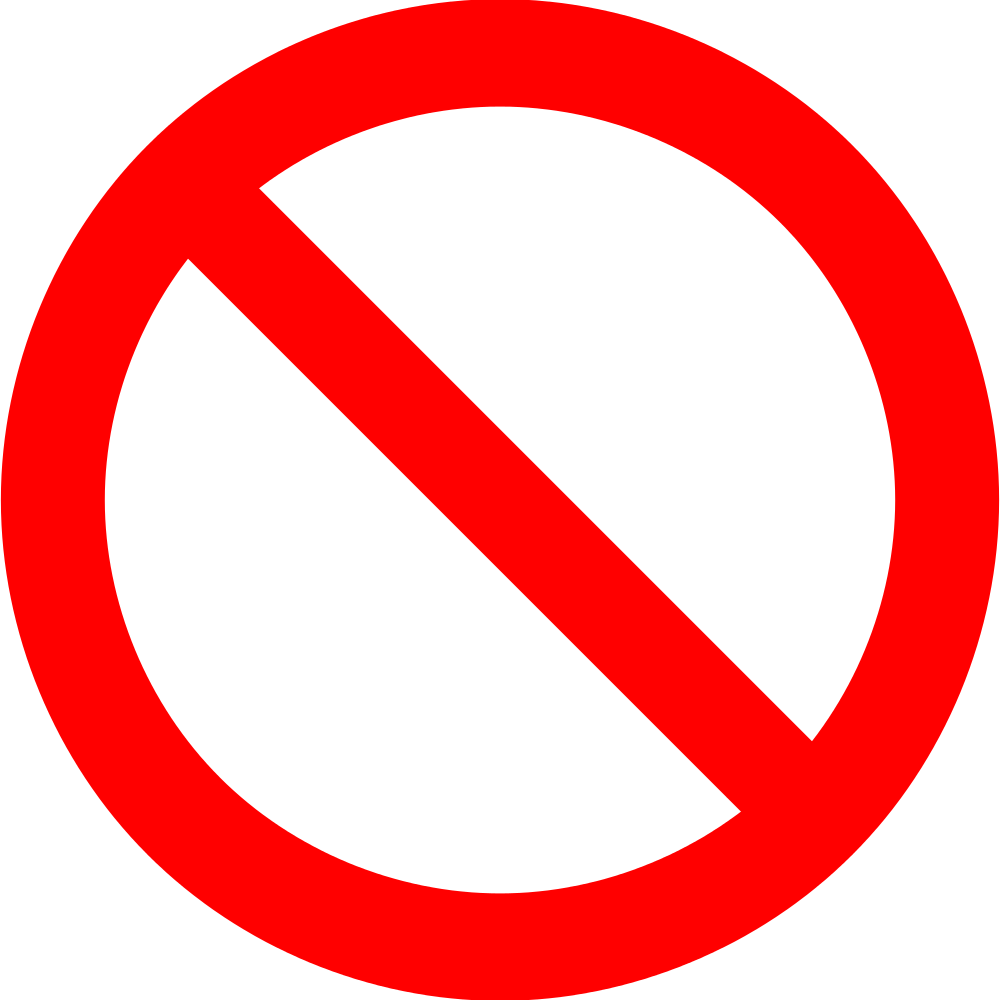
- Schoenegger, P., Park, P. S., Karger, E., & Tetlock, P. E. (2024). “AI-Augmented Predictions: LLM Assistants Improve Human Forecasting Accuracy.” arXiv preprint arXiv:2402.07862.
Wednesday, March 6: Predicting the future of advanced AI (Arvind)
- Pre-read
- Jason Wei, Yi Tay, Rishi Bommasani, Colin Raffel, Barret Zoph, Sebastian Borgeaud, Dani Yogatama, Maarten Bosma, Denny Zhou, Donald Metzler, Ed H. Chi, Tatsunori Hashimoto, Oriol Vinyals, Percy Liang, Jeff Dean, William Fedus (2022). “Emergent Abilities of Large Language Models”. arXiv.
- Ezra Karger, Josh Rosenberg, Zachary Jacobs, Molly Hickman, Rose Hadshar, Kayla Gamin, Taylor Smith, Bridget Williams, Tegan McCaslin, Stephen Thomas, Philip E. Tetlock (2023) “Forecasting Existential Risks: Evidence from a Long-Run Forecasting Tournament”. Read the first 4 sections and the AI subsection of Section 5.
- Clark R. Chapman, David Morrison (1994). “Impacts on the Earth by asteroids and comets: assessing the hazard”. Nature 367, 33–40.
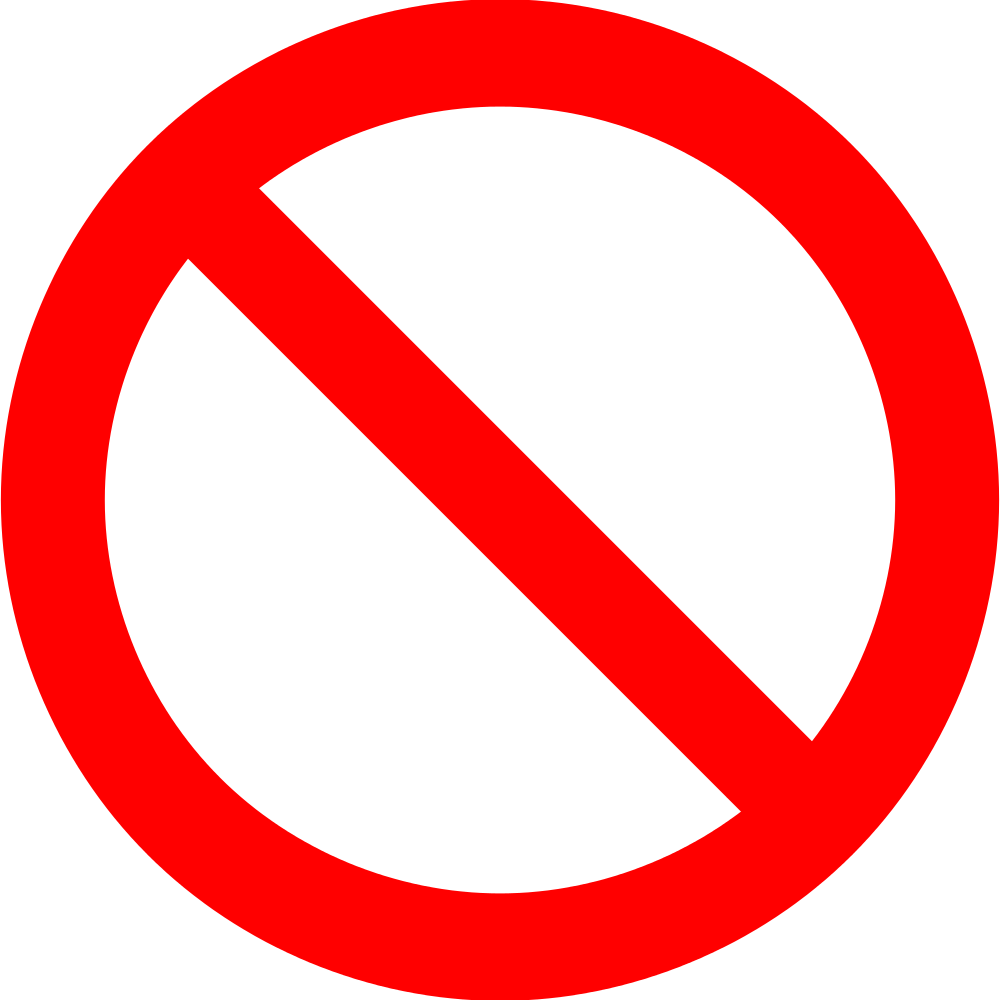
- Sayash Kapoor, Arvind Narayanan (2023). “AI and existential risk: a fork in the road”. Available to enrolled students on Ed.
Spring break
Monday, March 18: Life trajectories, part 1 (Matt)
Wednesday, March 20: Life trajectories, part 2 (Matt)
- Pre-read
- Lundberg et al. (2023) “The origins of unpredictability in life trajectory prediction tasks”. (Version we will read for class is posted on Ed). (Public working paper: https://arxiv.org/abs/2310.12871)
- 4 in-depth interviews (available from the instructor)
Monday, March 25: Measuring predictability (Arvind)
Wednesday, March 27: Uncertainty about uncertainty: Ideas from national security, prediction markets, and climate science (Matt)
Monday, April 1: Common pitfalls (Arvind)
- Pre-read
- Kapoor, S., & Narayanan, A. (2023). Leakage and the reproducibility crisis in machine-learning-based science. Patterns, 4(9). Elsevier.
- Kapoor, S., Cantrell, E., Peng, K., Pham, T. H., Bail, C. A., Gundersen, O. E., Hofman, J. M., Hullman, J., Lones, M. A., Malik, M. M., et al. (2023). Reforms: Reporting standards for machine learning based science. arXiv preprint arXiv:2308.07832.
- Hofman et al. (2017) “Prediction and Explanation in Social Systems”
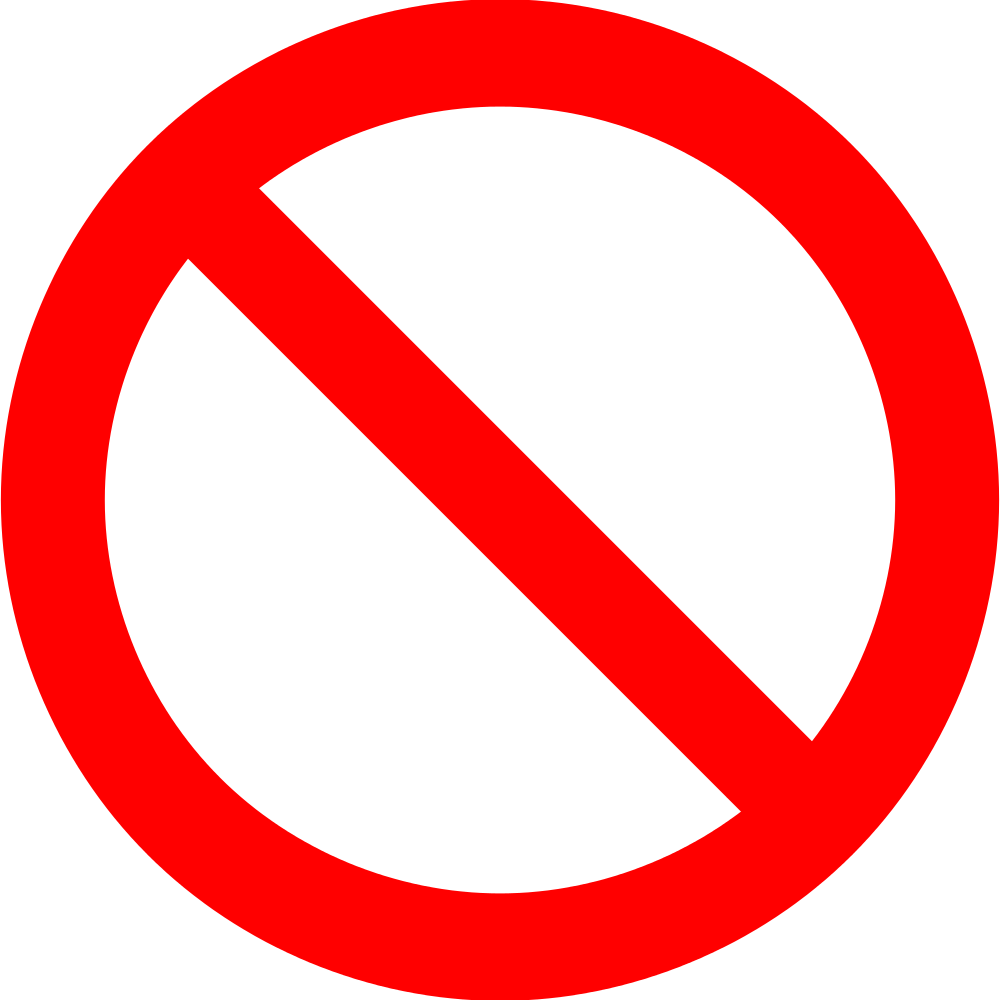
- Lipton, Z. C., & Steinhardt, J. (2018) Troubling Trends in Machine Learning Scholarship.
- Sainz, O., Campos, J., García-Ferrero, I., Etxaniz, J., de Lacalle, O. L., & Agirre, E. (2023). NLP Evaluation in trouble: On the Need to Measure LLM Data Contamination for each Benchmark. In Findings of the Association for Computational Linguistics: EMNLP 2023 (pp. 10776-10787).
Wednesday, April 3: Benchmarks (Arvind)
- Pre-read
- Marc Liberman (2015) “Reproducible Research and the Common Task Method”, Simons Foundation Lecture.
- Solon Barocas, Moritz Hardt, and Arvind Narayanan (2023). Fairness and Machine Learning: Limitations and Opportunities, MIT Press. Chapter 8: “Datasets”
- Josh Gardner, Zoran Popovic, Ludwig Schmidt (2024). “Benchmarking Distribution Shift in Tabular Data with TableShift”, Advances in Neural Information Processing Systems, Volume 36.
- Jake M. Hofman, Angelos Chatzimparmpas, Amit Sharma, Duncan J. Watts, Jessica Hullman (2023). “Pre-registration for Predictive Modeling”, arXiv.
Monday, April 8: Second taming of chance? Predictions about individuals and populations (Matt)
Wednesday, April 10: What’s your West Antarctic Iceberg? Isolating sources of uncertainty (Matt)
Monday, April 15: Killing Laplace’s Demon (Matt)
Wednesday, April 17: Strategic behavior (Arvind)
- Pre-read
- Chadefaux, Thomas (2017). “Conflict forecasting and its limits”, Data Science, 1(1-2), 7–17.
- Arvind Narayanan, Sayash Kapoor (2024). “Why is it hard to predict pandemics?” (Available on Ed.)
- Bambauer, Jane and Zarsky, Tal (2018). “The algorithm game”, Notre Dame L. Rev., 94, 1. Part 1 only.
- Zou, Andy and Wang, Zifan and Kolter, J Zico and Fredrikson, Matt (2023). “Universal and transferable adversarial attacks on aligned language models”, arXiv preprint arXiv:2307.15043.
- Manheim, David and Garrabrant, Scott (2018). “Categorizing variants of Goodhart’s Law”, arXiv preprint arXiv:1803.04585.
Monday, April 22: Time (Arvind)
No readings.
Wednesday, April 24: The future is open (Matt)
No readings.